Gas Distribution Mapping
Gas distribution modeling (GDM), also referred to as gas distribution modeling, is the process of deriving a truthful representation of how gases are dispersed in an environment from a set of spatially and temporally distributed measurements of relevant variables, like the gas concentration or the wind vector.
GDM constitutes an ideal application area for mobile robotics since they can provide an adaptive resolution of the distribution model while still offering the required accurate localization of each measurement. Installing sensors on mobile robots allows the creation of gas distribution models on the fly, thus the possibility of making decisions upon such a model, as for example, what locations to explore next.
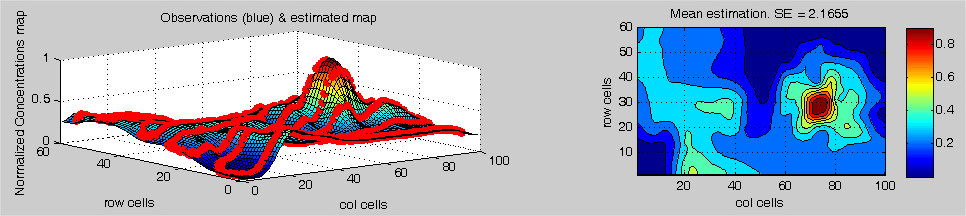
Building GDM with a mobile robot is a challenging task, mainly because in many realistic scenarios gas is dispersed by turbulent advection, which creates packets of gas that follow chaotic trajectories. This means that the gas distribution is, in general, not static but is continuously changing instead. Despite these problems, GDM has important applications in industry, science, and every-day life. Mobile robots equipped with gas sensors are deployed, for example, for pollution monitoring in public areas, surveillance of industrial facilities producing harmful gases, or for the inspection of contaminated areas within rescue missions.